Table of Contents
Data Deep Dive: The Origins of and Statistical Models Underpinning FOUNT’s Use of Data
The difference between FOUNT and other employee feedback collection methods (interviews, focus groups, tool usage data, process mining, and etc.) is as big as the difference between what work feels like and how work actually gets done. This article breaks down how our model provides more valuable data by focusing on: a) Impact over volume b) Specific work moments over general work experience c) Actual problems to solve over broad ideas AI transformations are built on data. Find out why work friction data should be your first choice as a leading indicator of ROI.
Your organization is about to undergo a costly AI transformation. You’re looking for some data-backed insight to guide the deployment and help you reach your ROI targets. And you need to get other leaders on board with your vision for the project. Welcome to FOUNT.
FOUNT is different from traditional employee experience surveys, which tend to question respondents across an entire organization with the goal of learning how they feel about their work. We’re interested instead in getting feedback about how that work functions.
Broad employee experience is certainly worth understanding, of course, but it’s the more specific data that FOUNT is after that can help facilitate something like an AI transformation. In this piece, we’ll explain how our unique statistical model came to be and show how it provides actionable recommendations for overcoming workplace obstacles and helping AI transformations become ROI positive.
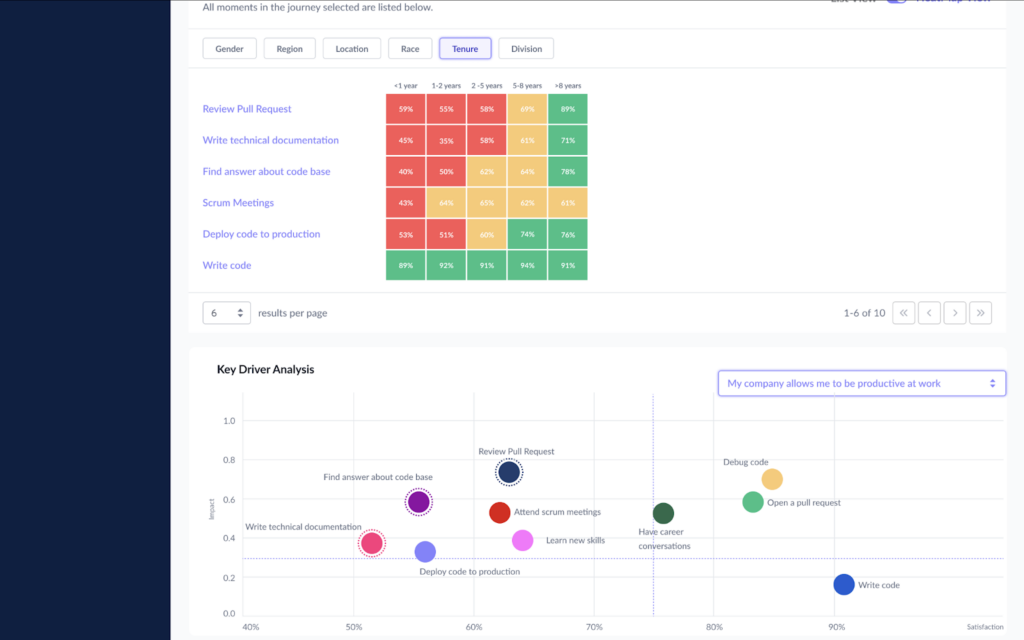
What We Gather: Enough Data Points to Identify Moments of Work Friction
FOUNT’s statistical model was initially developed to track an employee’s journey through their lifecycle with an organization. The goal was to determine which work moments mattered the most to the employer’s ability to attract and retain talent; that is, those that had the most impact on Employee Net Promoter Scores (eNPS). To get there, we ask employees work-specific and role-specific questions.
For example, if we’re surveying a call center team, our questions will be aimed directly at the type of work they do every day. How do they prioritize customer calls? Where do they seek out the information they need to answer various questions? How do the technologies, processes, and people involved impact that work? What issues do they run into in trying to complete their tasks?
What these data points end up measuring – and what constitutes the focus of the FOUNT approach – is work friction. By isolating the moments in employees’ work days that slow them down or cause them trouble, we’re moving past general feelings about work and drilling down into role-specific issues.
This targeted approach means we don’t need the huge volume of general responses that other employee surveys demand. Our surveys gather data related to specific tasks and roles within an organization – and therefore from a much smaller pool of respondents. In fact, FOUNT can find statistical relevance in a sample size of as little as 53 responses on any given moment (though we often take more into account).
How We Parse It: Data Analysis that Measures Satisfaction and Impact
Fewer responses doesn’t mean less insight – in fact, just the opposite. FOUNT isn’t trying to determine how most or all employees feel about their work. We’re looking for insights into what is and isn’t working within their specific tasks.
We get to that by asking role-related questions about those tasks, with workers providing a grade (on a five-point scale) of how satisfied they are with each of those moments. In this way, we’re actually measuring work itself through a first-person lens to determine which specific employee moments are most relevant and most impactful.
For example, an HR professional may have to terminate employees from time to time. Not surprisingly, this unpleasant responsibility would likely earn low satisfaction ratings. On the other hand, however, they’re probably not going to quit because of it – it’s just part of the job. There’s a distinction between the satisfaction (low) and the impact (low) of the task.
This method can provide more nuance as well. For example, we surveyed a team of software developers on their interaction with a new generative AI tool across several daily work activities. The results showed that the more experienced developers had high ratings for the tool, while the more junior team members reported much lower satisfaction.
In this case, that data divide didn’t just reveal how workers felt about the tool – it offered a potential way to make it more useful for the team. Instead of scrapping the project based on negative feedback from the less-experienced developers, the firm instead provided additional training to this group. The result was a retooled rollout that saved the investment and resulted in more than $5 million in annual cost savings.
This success story, and many others like it, demonstrate a key attribute of our approach – it doesn’t require full unanimity to diagnose any given work obstacle. Instead, we’re after a statistically relevant consensus among a targeted group of employees.
How it Helps You: Clear Insight into Problem Areas so You Can Adjust
Getting clear data on what’s working and what’s not shows an organization which specific problems need the most urgent attention so it can better know where to focus its efforts. This is why work friction analysis is particularly helpful in AI transformations:
- Focus: Work friction data can help single out where in the organization an AI tool should best be deployed or how it can be reconfigured to increase productivity.
- Adjust: Ongoing analysis of that data can provide insight into how an AI rollout is performing – that is, how employees are adopting and interacting with the tool – which an organization can use to make adjustments, if needed.
Any AI project is going to bring with it the kind of significant costs and pressure that demand confident, data-backed decisions. Work friction analysis delivers actionable insights to fuel exactly those kinds of decisions.
The Power of Decision-Ready Insights
Traditional employee experience surveys can help you understand how employees feel about their work. But they don’t assess the actual work those employees do and where they specifically encounter problems. Work friction is next-level employee data that focuses on the actual work being done – which is where AI deployments live.
Getting to decision-ready insights is all about the how: By understanding how employees work – rather than just how they feel about that work – organizations can understand how AI can address the issues they’re having. So, when the decision-makers in your company want to know why you’re using work friction data to guide your AI project, you can explain just how important it is to success.
Get in touch to learn more.
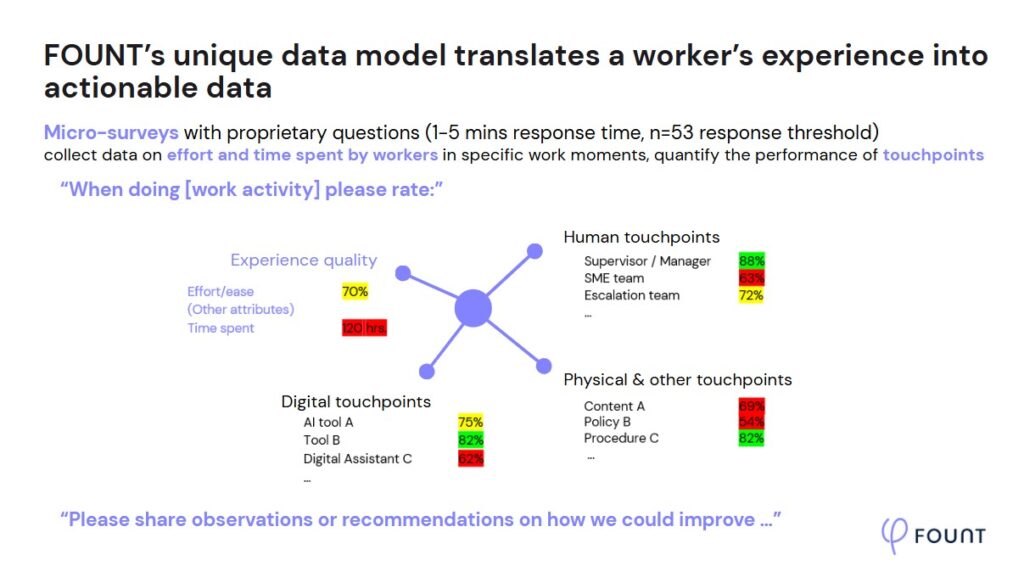
Related Resources
See all News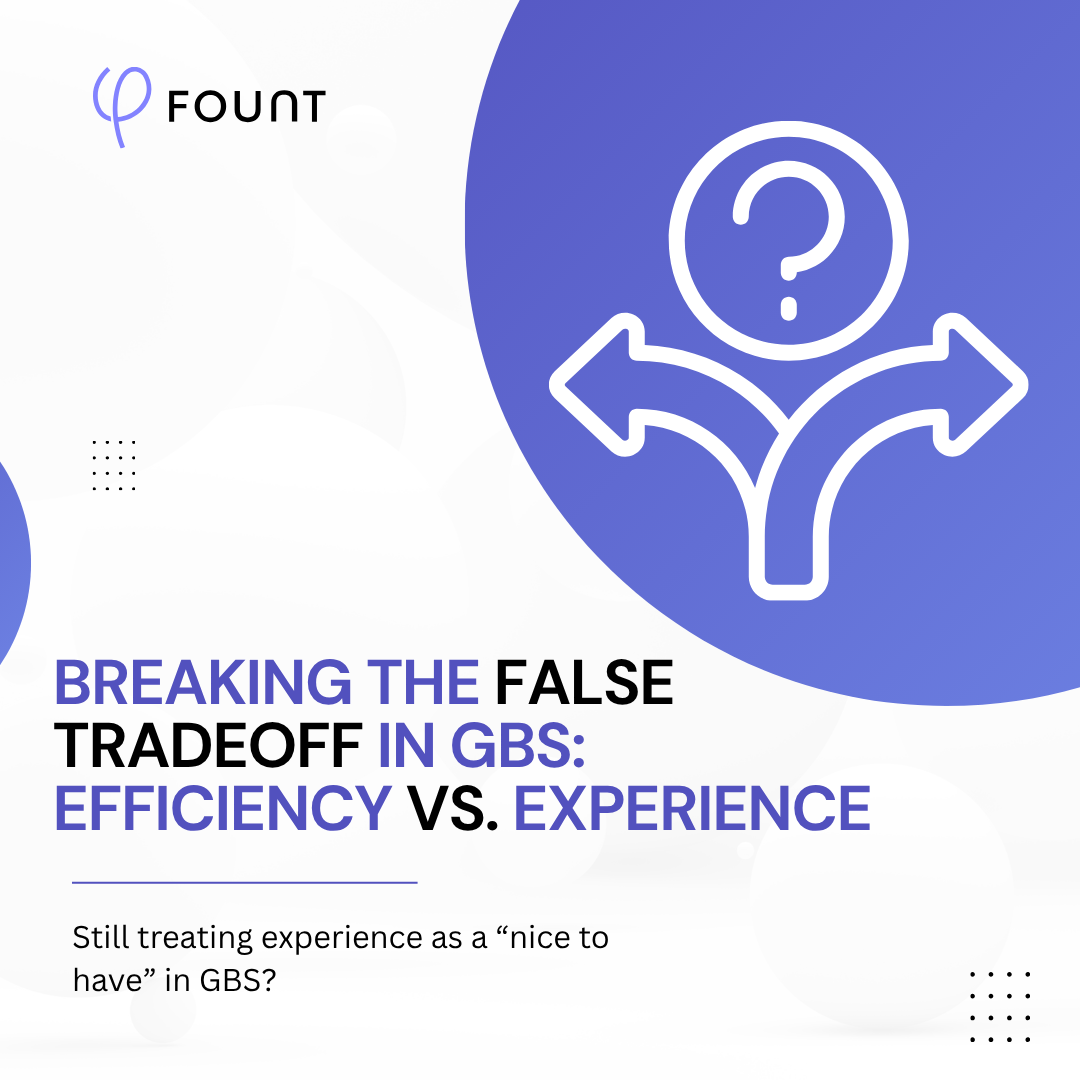
Insights
Breaking the False Tradeoff in GBS: Efficiency vs. Experience
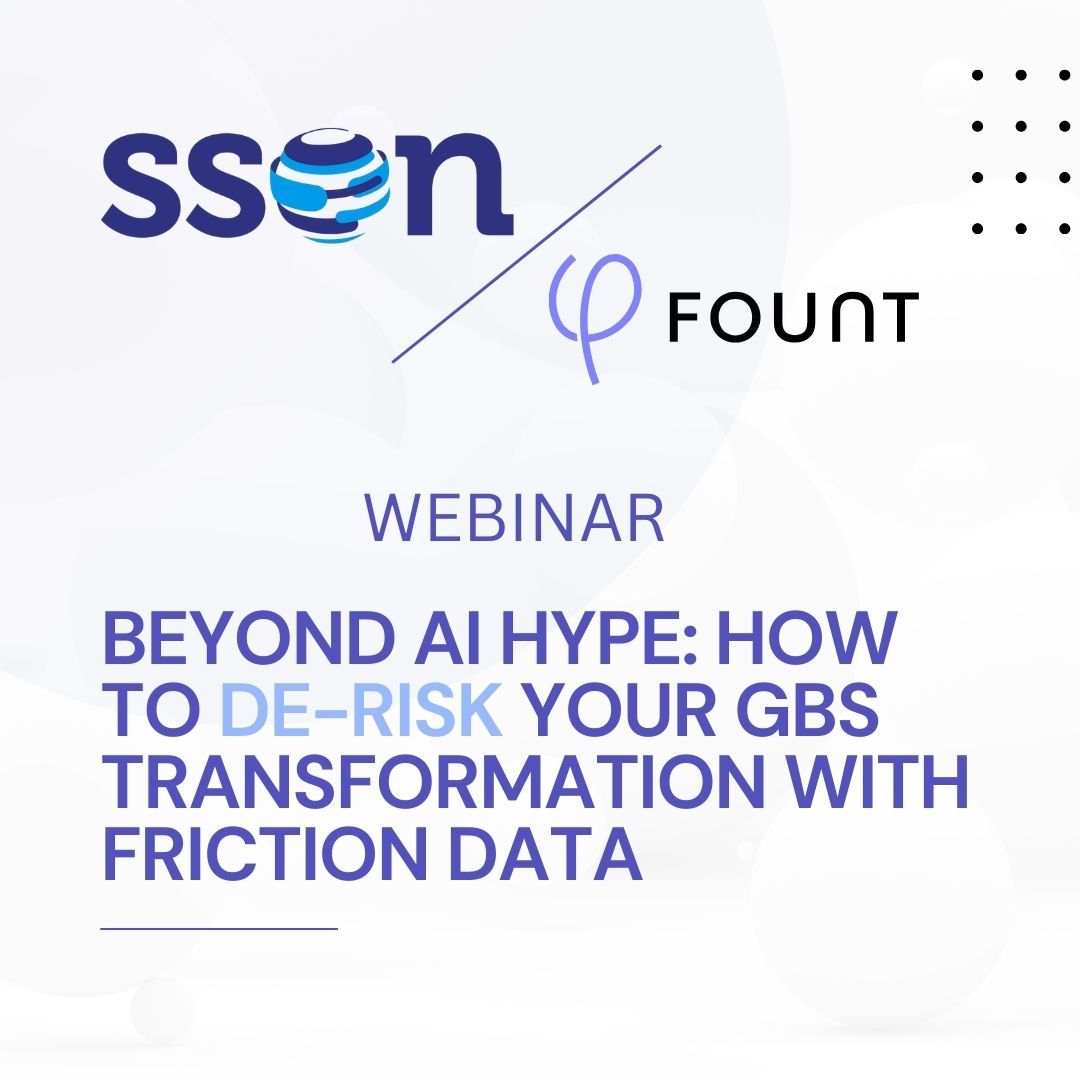
Events
LIVE Webinar – July 9th for SSON Network. Beyond AI Hype: How to De-Risk Your GBS Transformation with Friction Data
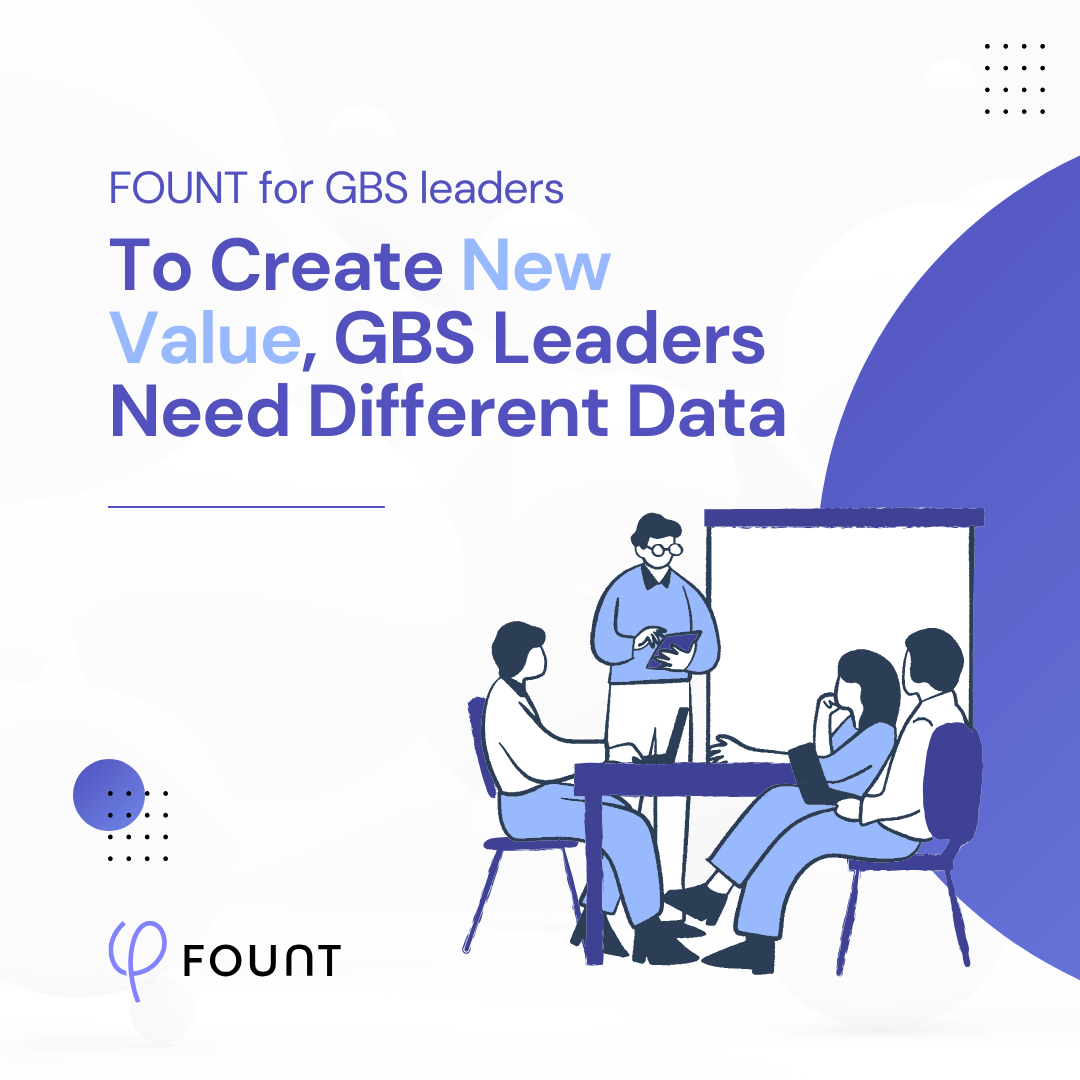
Insights
To Create New Value, GBS Leaders Need Different Data
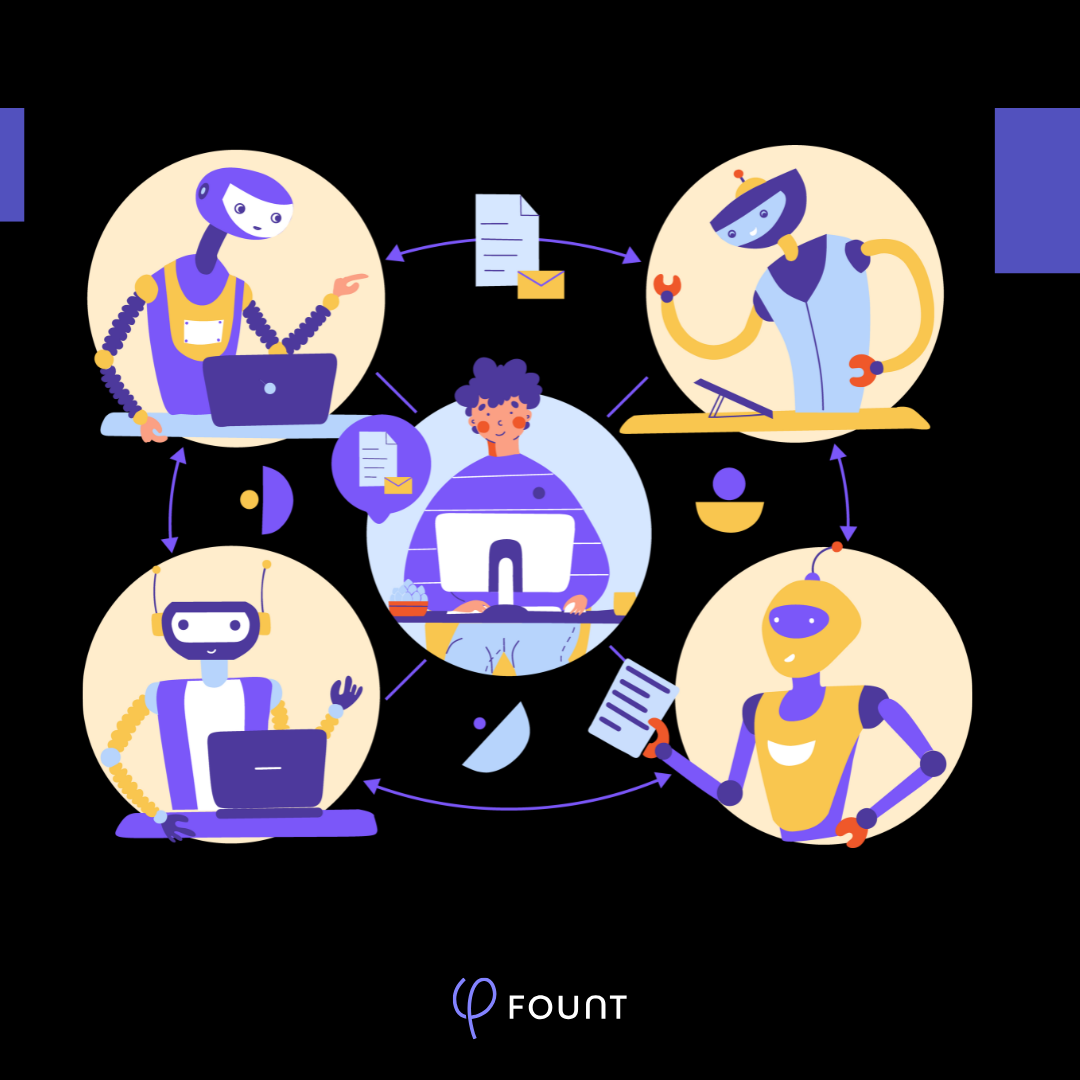
Insights
How to Keep Up with the Latest AI Developments
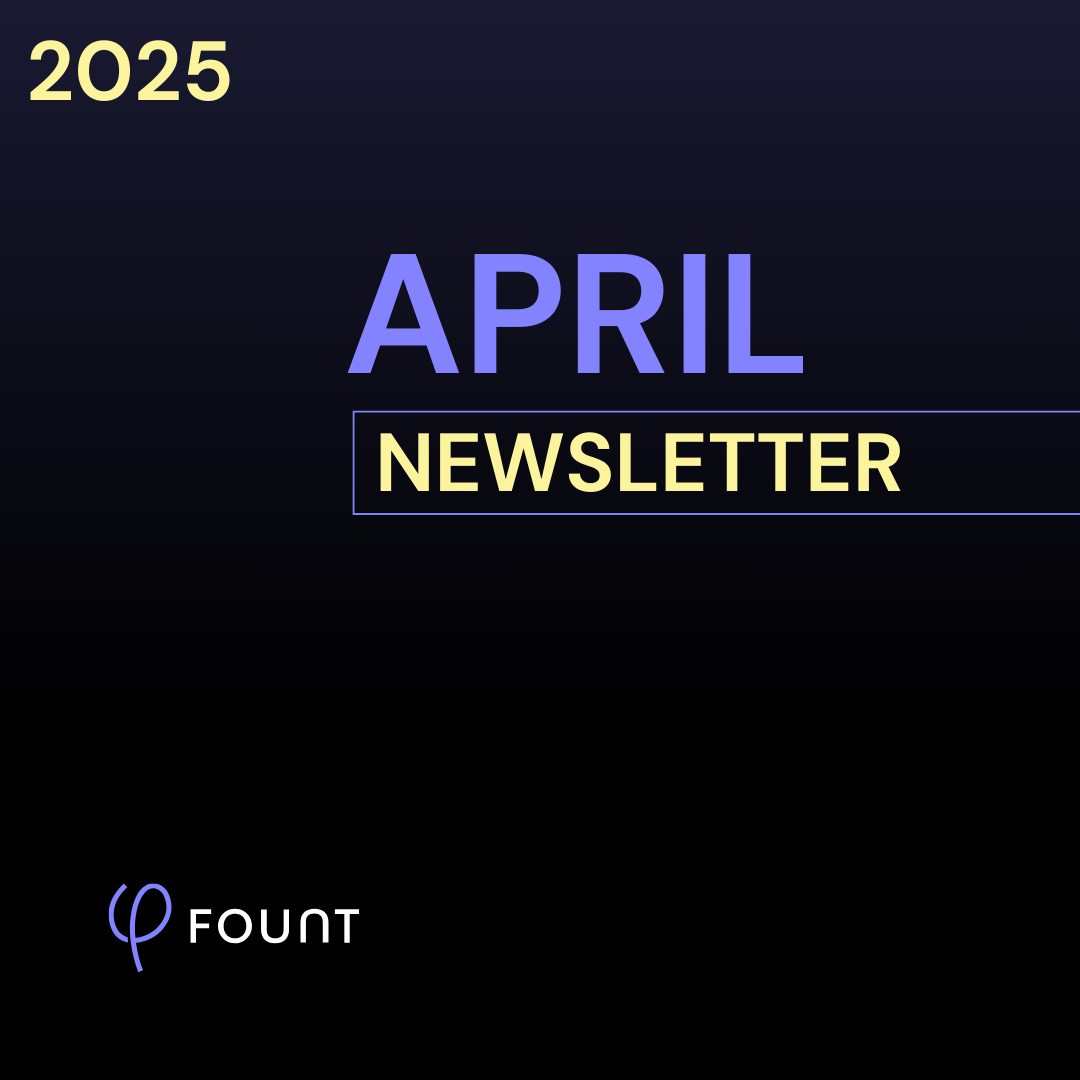
Insights
APRIL Newsletter. Friction: You Can’t Improve What You Can’t See
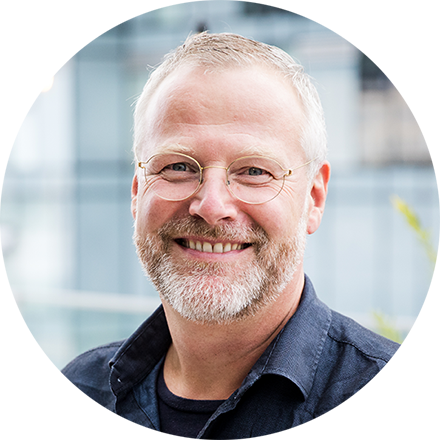
Guest Post
AI is Reshaping the HR Operating Model: Here’s What 15 Leading Companies Discovered
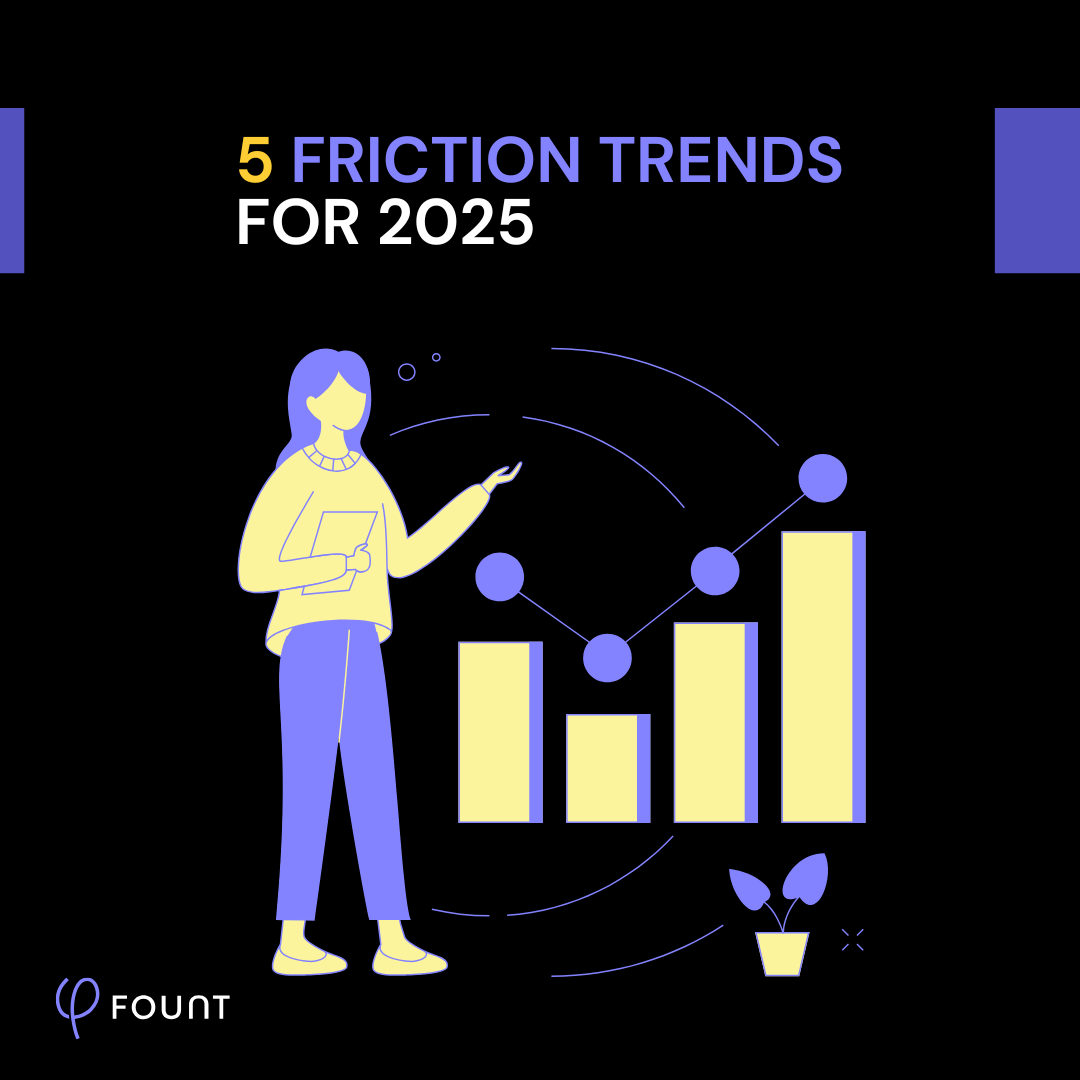
Insights
5 Friction Trends for 2025
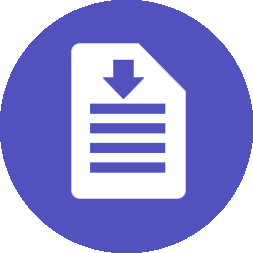
Research
WHITEPAPER: Work Friction