Table of Contents
Nobody Wants to (Measure) Work Anymore: The Real Problem with AI Transformations
KEY TAKEAWAYS Measuring the success of an AI project means measuring work, but many organizations don’t know how. Traditional work measurement tools such as engagement surveys and process mining can provide useful information, but can’t offer a deeper understanding of how employees do their work. Work friction analysis can uncover the day-to-day employee pain points that an AI tool might be able to relieve.
While the growth of AI continues apace, so too does the technology’s ability to confound many organizations. To wit: Forbes projects an annual growth rate of nearly 40 percent in AI over the next six years, but 60 percent of leaders worry they lack a plan and vision to implement it in their companies. That’s called a disconnect.
It doesn’t help that AI transformations are notoriously difficult, as evidenced by their high rate of failure. These are complicated implementations, of course, that come with a lot of questions for the organizations pursuing them: Where should we deploy AI? Which tools make sense? How will we measure success?
But it’s not necessarily the cost or the complexity of the technology itself that’s to blame for so many AI flameouts. The culprit is often the fact that measuring the success of an AI project means measuring work.
It’s simple, really – the goal of AI is to increase productivity and efficiency. And the basis of both productivity and efficiency is work. But most organizations don’t measure how the work their employees are doing is getting done.
In this piece, we’ll explain why it’s so crucial to measure work – especially when it comes time to deploy AI. Just as importantly, we’ll show you the right way to go about it.
Traditional Work Measurement Tools Aren’t Enough
Many organizations don’t measure the work their employees are doing on a day-to-day basis.
Engagement surveys, for example, can be great for determining how employees feel about their work. That’s good feedback to have! But they don’t provide a detailed understanding of how that work actually gets done or where problems tend to arise.
Similarly, process mining can be effective at uncovering operational inefficiencies in an organization. But it doesn’t provide information about, say, the ease of getting manager approvals or whether headsets are generally working. It doesn’t offer any insight into the employee perspective as to whether a proposed solution might actually solve the issues at hand.
In other words, engagement surveys and process mining both have their uses. But for something that requires a deep understanding of how work gets done – such as determining where to most effectively deploy an AI tool – you need to go further.
Measuring Work Means Examining Employee Touchpoints
In most instances, the purpose of AI is to increase productivity by removing obstacles and accelerating work. AI is meant to be a problem-solver, which is why you need to know what problem you want the technology to solve before you choose a tool or make an investment.
This is why measuring work is such an important step in any AI project. Only by understanding exactly where employees are experiencing work friction – the day-to-day pain points that hinder their productivity – can you begin to understand how AI can help.
FOUNT does this by surveying workers about individual moments in their days (that is, specific tasks they complete) and specific touchpoints involved in those moments (including people, processes, and technology involved in completing a task).
We ask about the impact of various moments and touchpoints, as well as workers’ satisfaction with each. When we find areas with high impact and low satisfaction, we know we’ve hit on work friction. To date, we’ve identified more than 8.5 million friction points for our clients.
Understanding where the friction lies in your organization is powerful because friction points are where AI tends to be most effective. For example, work friction analysis could pinpoint a moment in a development workflow – say, reviewing pull requests – where team members could use an AI tool to complete their work more quickly. No more guesswork.
AI Success Depends on Employee Buy-in
When you’ve identified high-friction work moments that AI can improve, you’re well on your way to adopting AI in an ROI-positive way. But it’s important to note that you can’t just take your work friction data and proceed with AI as if it were any other digital transformation.
AI transformations instead require a bottom-up approach. Employees must see the value of the tool for themselves in their work and therefore willingly adopt and use it.
So getting employee input shouldn’t be a one-and-done proposition. Survey them to identify initial friction points, then survey them to identify friction points after the initial implementation. Adjust settings, tweak workflows, and otherwise do what’s needed to make work work.
Efficiency and productivity are important metrics to track for board meetings, of course, but they’re lagging indicators. If you want those metrics to improve from one quarter to the next, it’s essential to measure work in the interim and address the areas preventing improvements – whether or not the fixes involve AI.
With AI, Measure Once, Then Cut
The continued growth of AI demands organizations to be ready and able to take advantage of the opportunities the technology affords – or risk falling behind. Having the information you need to make confident decisions about how and where to deploy is a good place to start.
AI may be high-tech, after all, but its success or failure in an organization tends to be rooted in the spirit of an age-old construction axiom: Measure twice, cut once. And when it comes to AI, even one measurement is better than nothing before jumping in with a big investment.
The key is to make sure you’re measuring the right things – not just how your employees feel about their work, but how they actually do their work. A deep understanding of the latter will show you how and where AI can be most effective. We can help show you how.
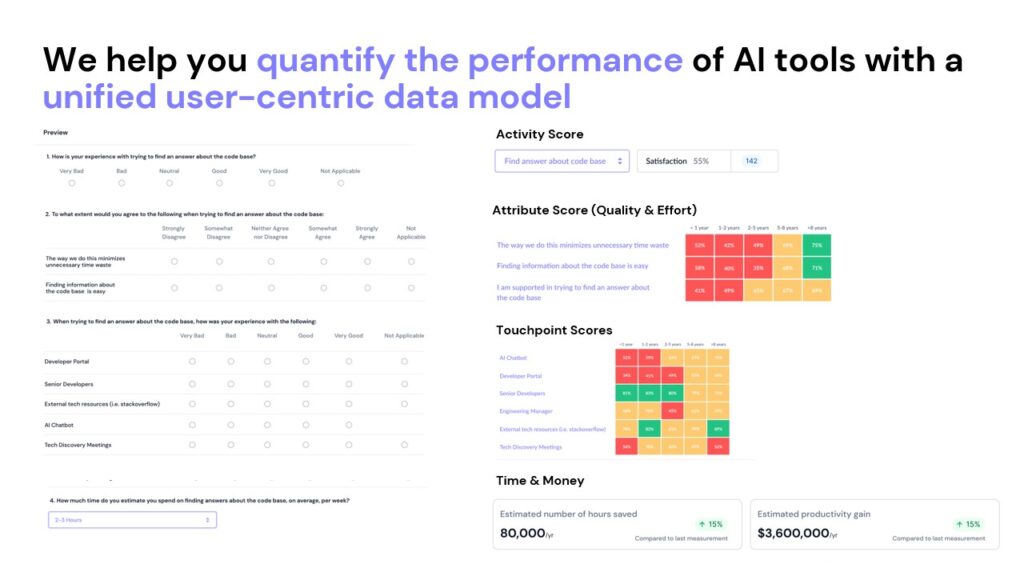
Related Resources
See all News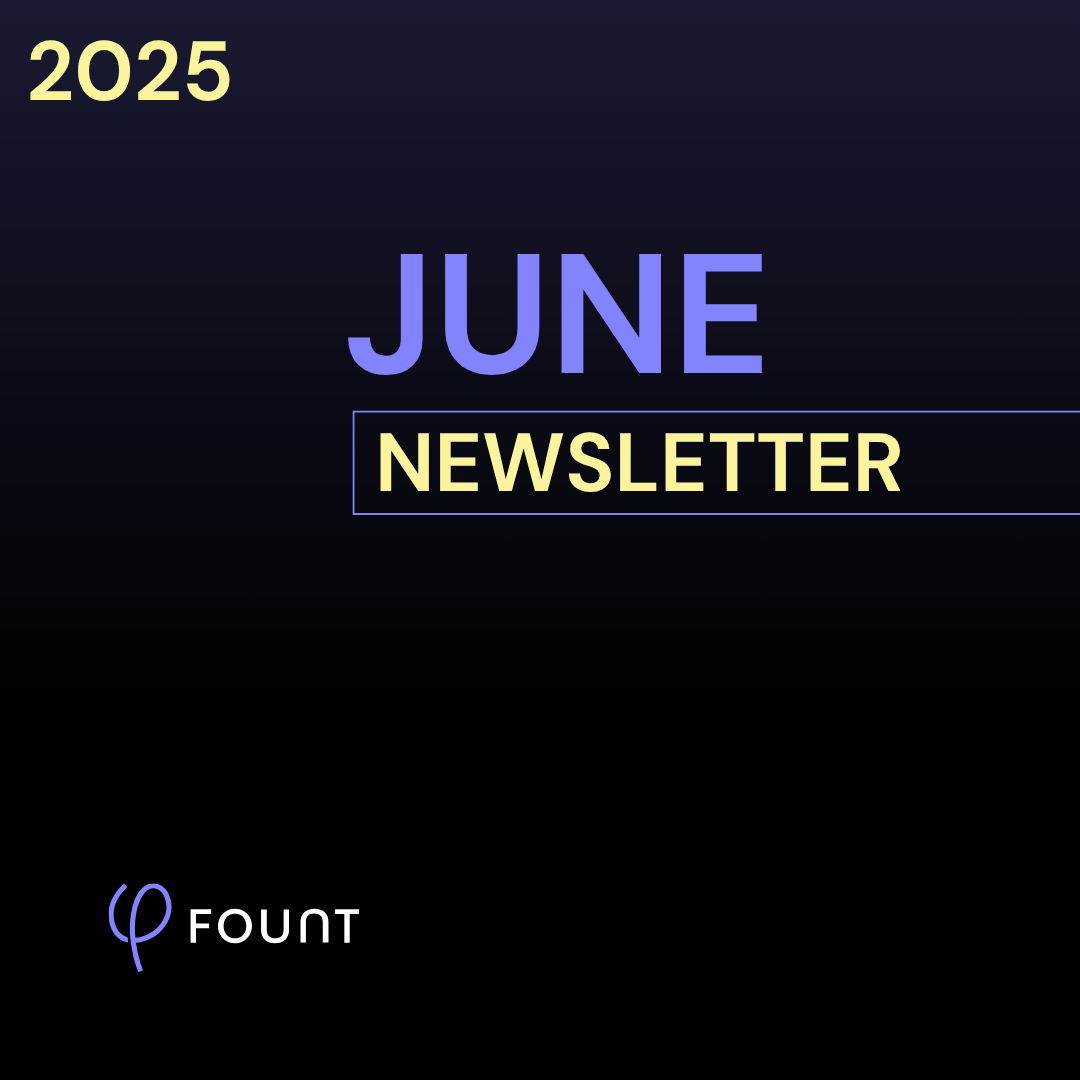
FOUNT News
June Newsletter: Friction is Killing Your AI ROI.
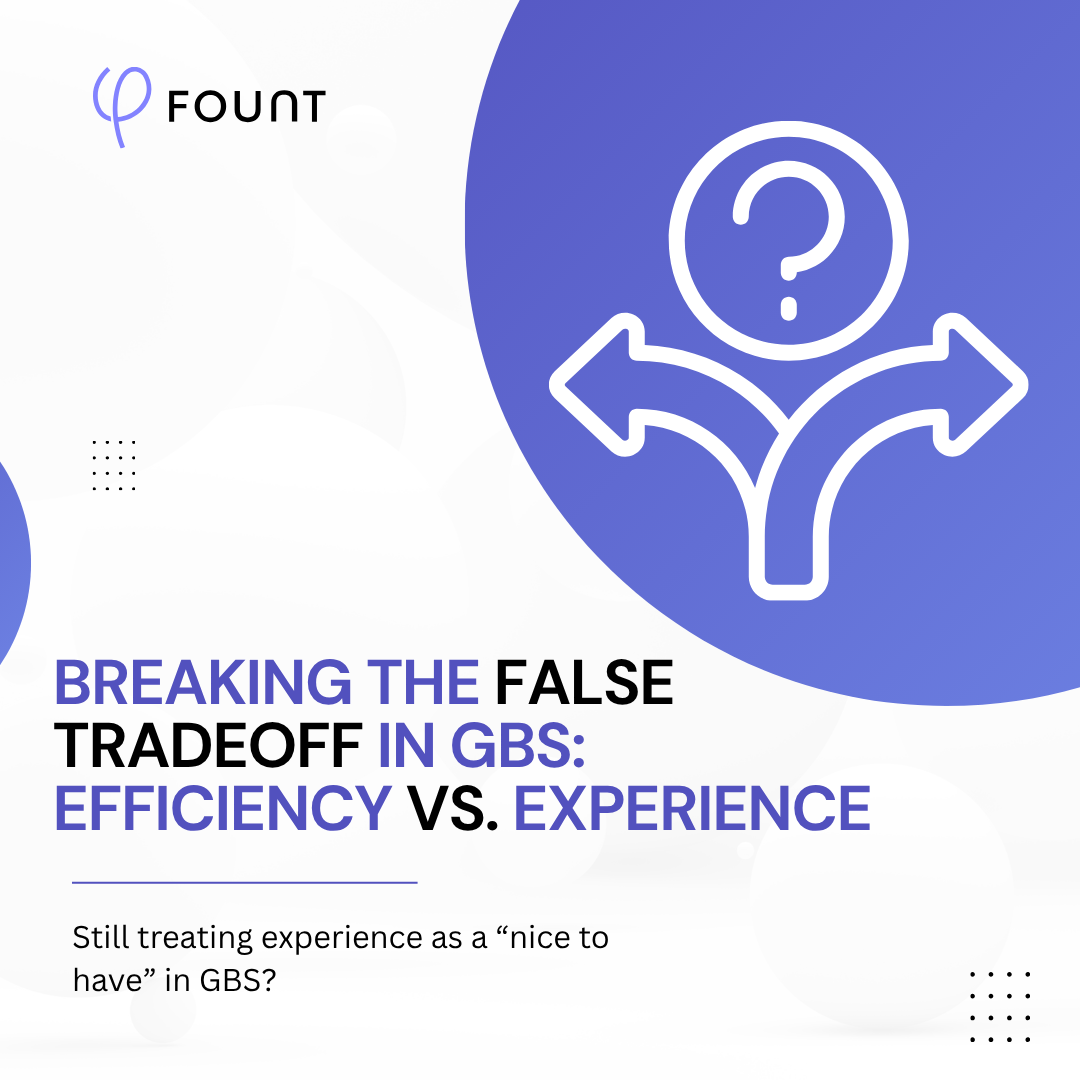
Insights
Breaking the False Tradeoff in GBS: Efficiency vs. Experience
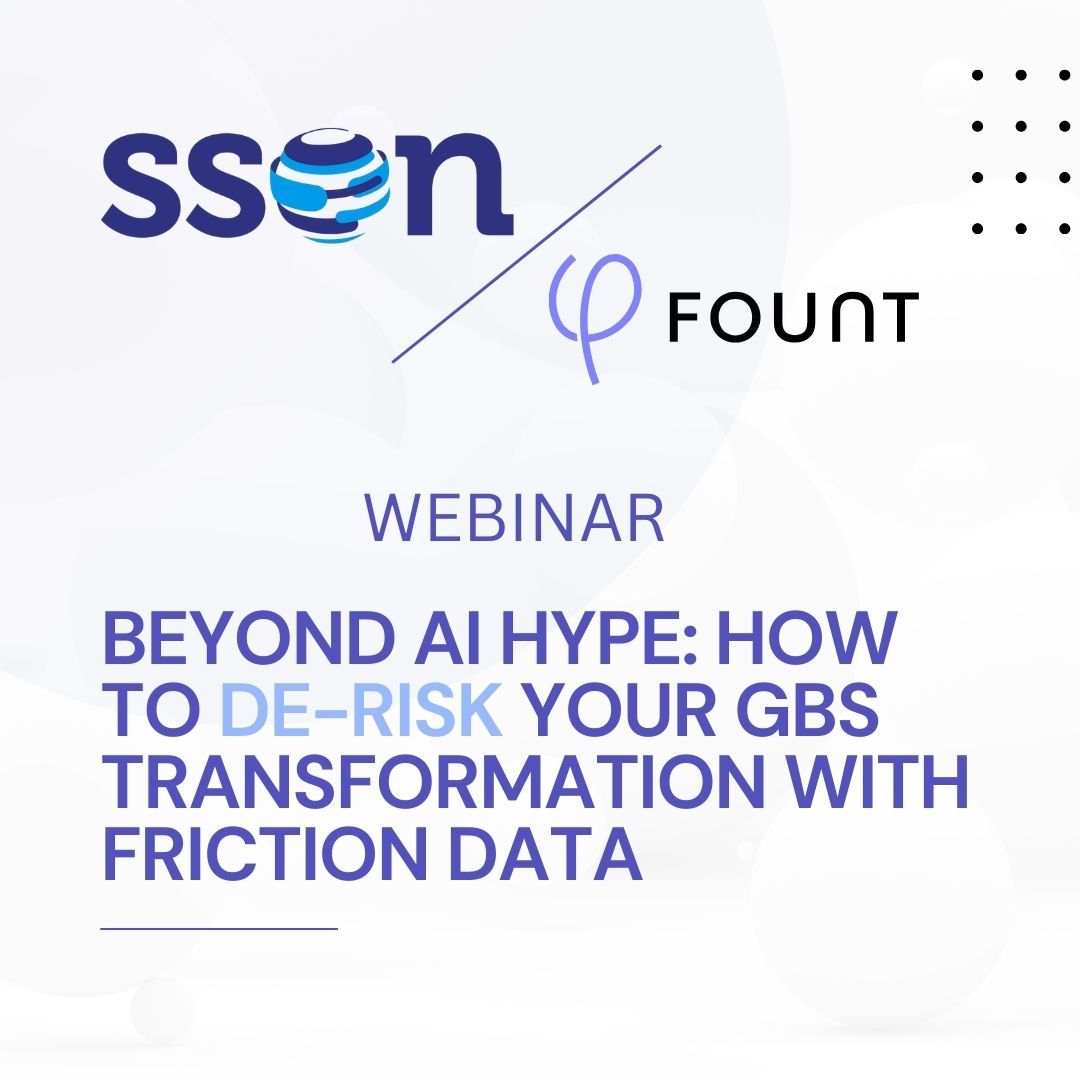
Events
LIVE Webinar – July 9th for SSON Network. Beyond AI Hype: How to De-Risk Your GBS Transformation with Friction Data
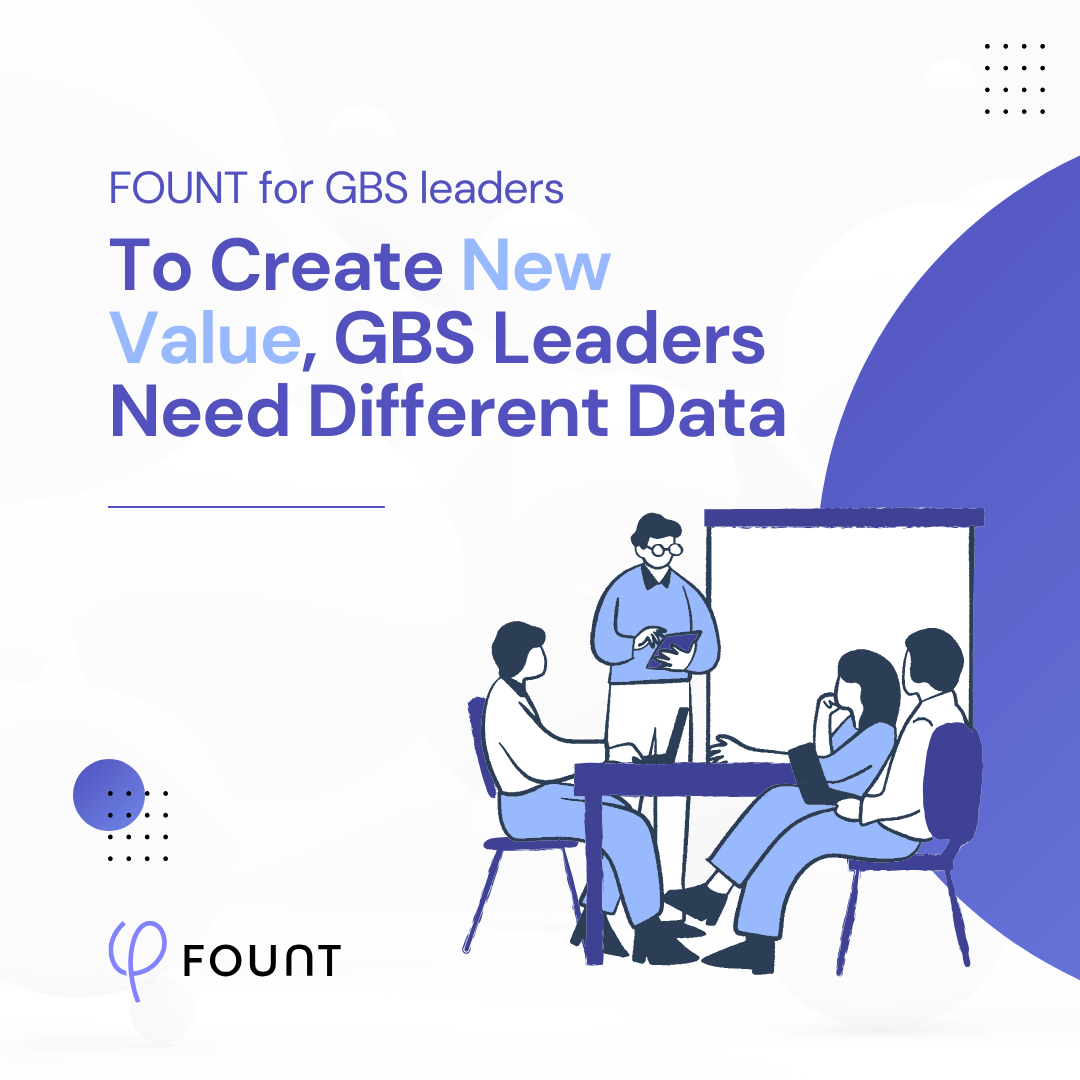
Insights
To Create New Value, GBS Leaders Need Different Data
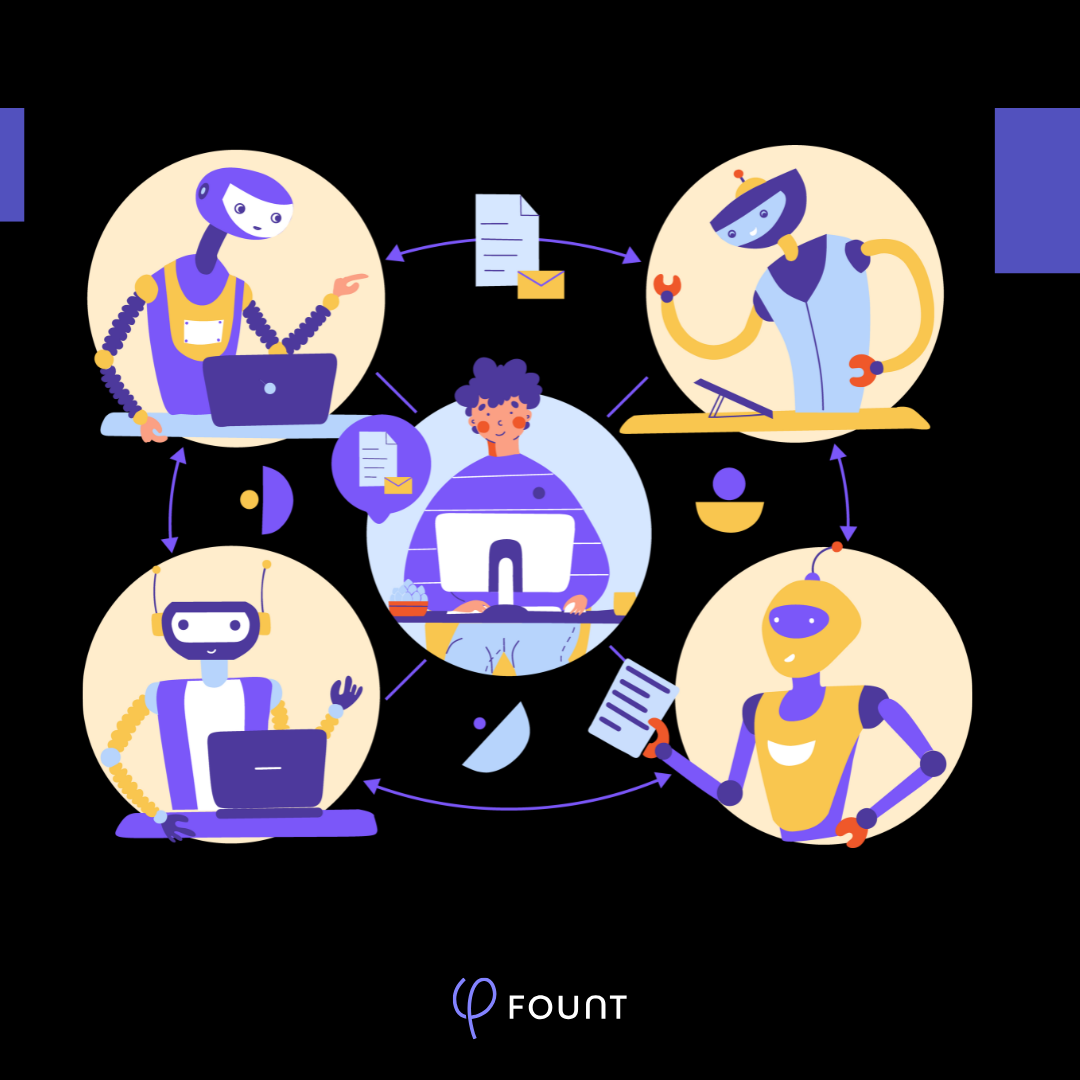
Insights
How to Keep Up with the Latest AI Developments
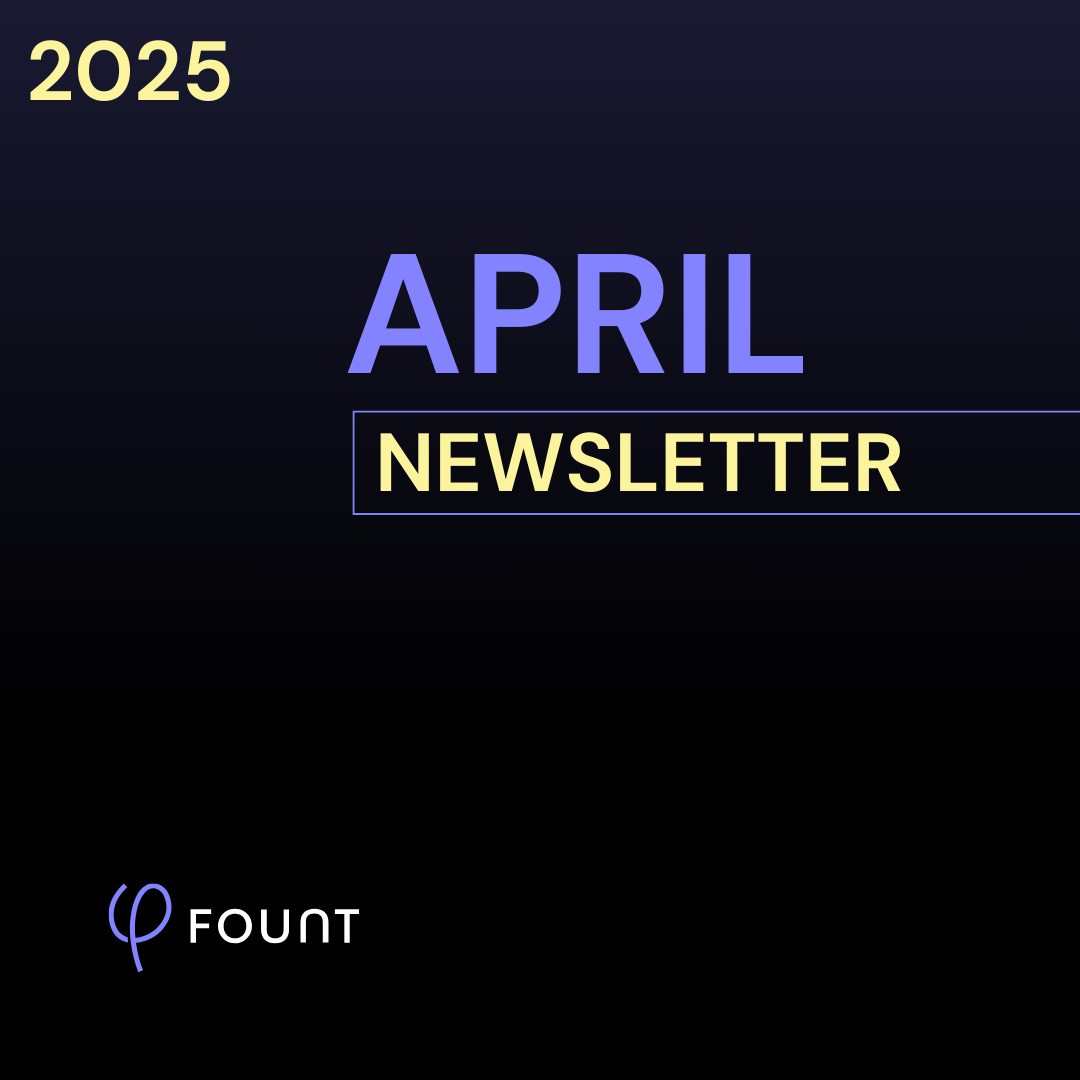
Insights
APRIL Newsletter. Friction: You Can’t Improve What You Can’t See
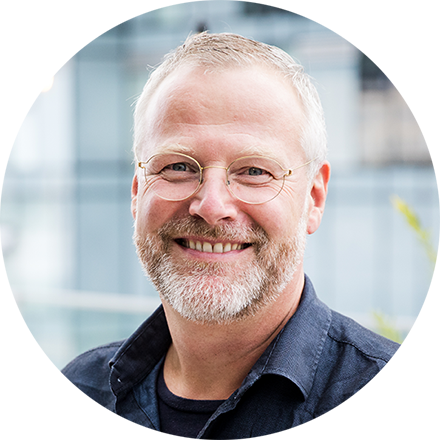
Guest Post
AI is Reshaping the HR Operating Model: Here’s What 15 Leading Companies Discovered
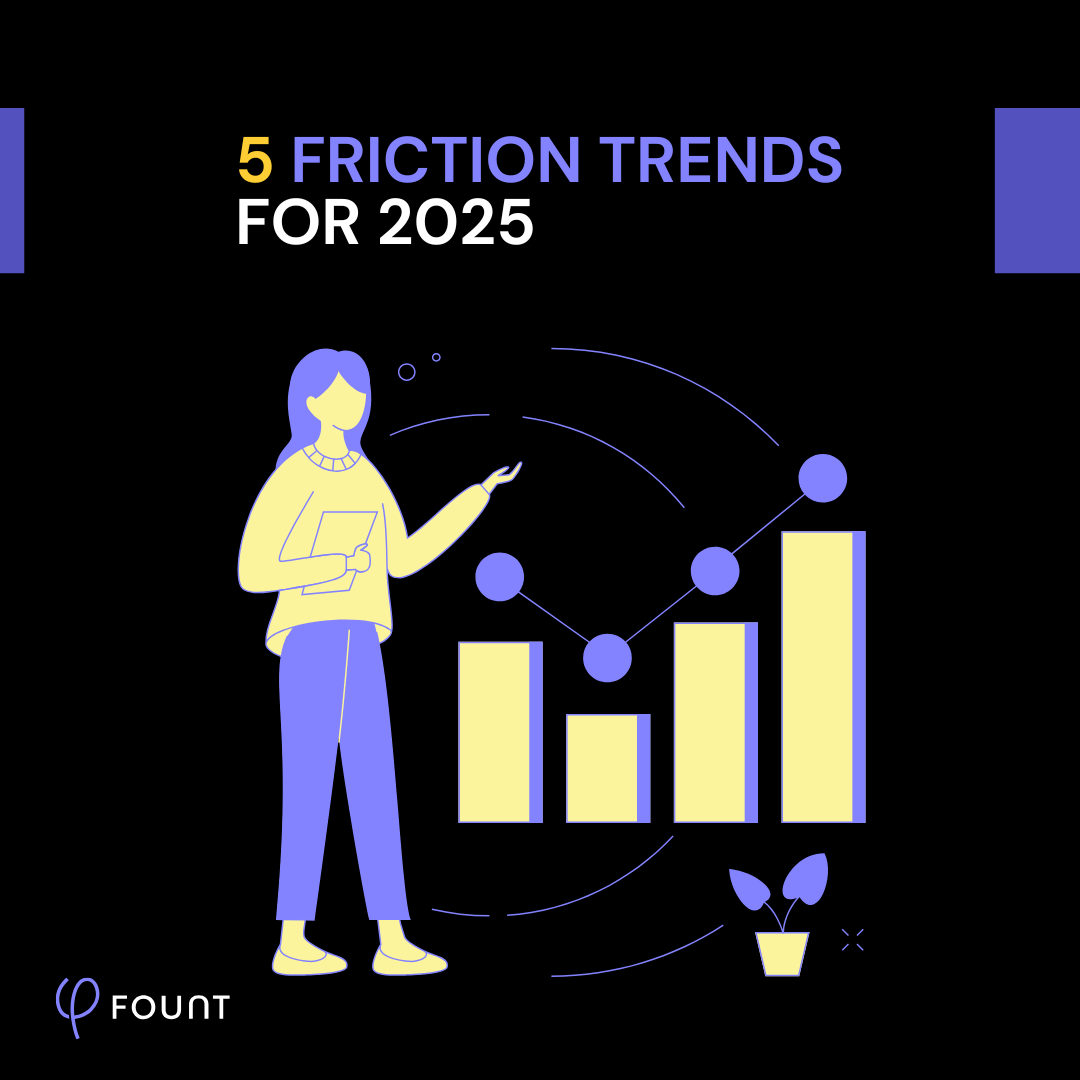
Insights
5 Friction Trends for 2025