AI Implementations Need Better Validation Metrics
Most AI projects struggle to prove ROI early enough to justify ongoing investment. Traditional metrics like efficiency and productivity gains often take years to materialize, which doesn’t align with leadership expectations. This article explains why measuring work friction - how work actually gets done and where it slows down - can serve as a powerful early indicator of whether AI tools are helping or hurting. By gathering real-time feedback on how AI impacts day-to-day tasks, leaders can validate AI implementations in weeks rather than years.
KEY TAKEAWAYS
- Determining ROI – especially early – continues to be one of the biggest challenges for organizations undertaking AI transformations.
- Metrics related to increased productivity or efficiency from AI can take years to materialize – and most organizations don’t have the patience to wait that long on such a big investment.
- Measuring AI’s impact on the specific work employees are doing provides a good leading indicator of a project’s potential success.
One of the biggest challenges for organizations looking to harness the power of AI continues to be justifying its cost. While promises of productivity and efficiency increases sound great, AI is ultimately an investment like any other: the sooner the technology shows ROI, the better.
That’s why this issue, more than any technical consideration, is what tends to impede AI progress in most organizations. Despite 85 percent of companies reporting progress in their AI strategy execution, only 47 percent say they’ve seen positive ROI from their AI implementations.
A big part of the problem is that it can take a long time – often several years – to get data on things like productivity and efficiency improvements associated with AI. And that time horizon often doesn’t align with that of the decision-makers funding these big investments: half of CFOs will kill an AI project without ROI after a year.
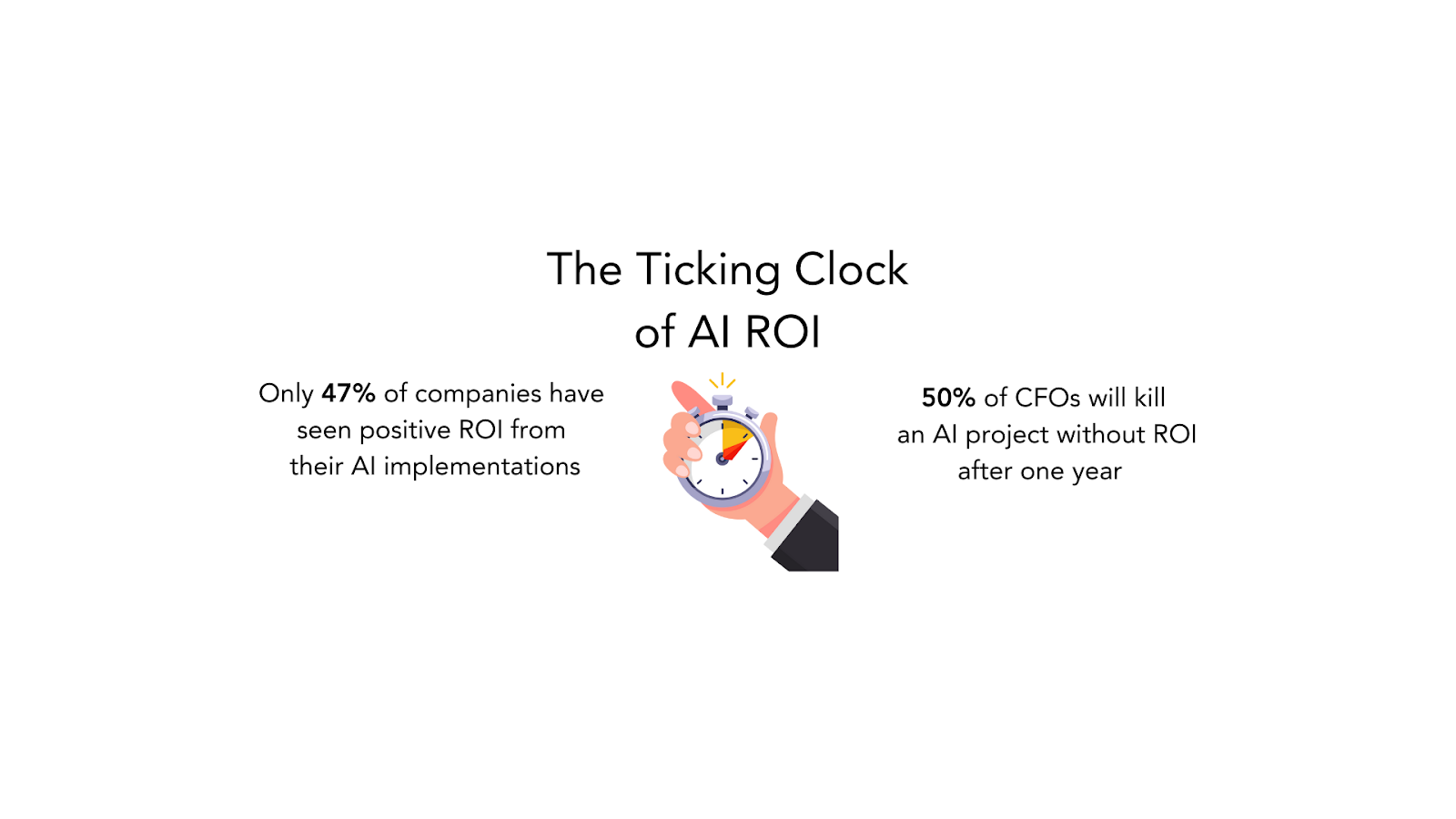
So while companies are excited about the possibilities of AI, most are far less certain they’ll be able to validate the technology and prove its impact – especially in those pressure-packed, make-or-break early stages. In this piece, we’ll explain why anyone looking for a leading indicator of AI success should be looking to their workforce.
What Type of Data Could Be a Leading Indicator of AI ROI?
As noted, most AI investments aim for increased productivity or efficiency – both of which are notoriously difficult to measure (especially in real time). An organization that could do so in some approximation of real time would have an excellent window into whether its AI tool was on track to deliver positive ROI.
To measure productivity, we need to be able to measure outputs vs. inputs. For example, work achieved against the people, time, money, energy, etc., needed to achieve that work:
- Did we do more with the same number of people or the same with fewer people?
- Did we increase our output with the same input costs or maintain our output while decreasing our inputs?
Likewise, when it comes to efficiency, we need to know not only whether things are getting done faster, but if the quality of work is slipping as a result of that uptick in speed:
- Did our people complete individual tasks faster?
- Did our teams get through cycles – sales cycles, product launch cycles, etc. – more quickly?
- Did we do things with fewer mistakes or less rework?
Most organizations, however, don’t have the systems in place to measure these types of things. Instead, they’re looking at…
- Higher-level metrics (qualified leads, sales pipelines, IT ticket completion metrics).
- Lagging metrics (turnover, quarterly revenue, task backlogs).
- Employee experience / sentiment analysis, which doesn’t speak to the impact of an AI tool on the actual work.
For Meaningful AI Data, Ask Your Workforce The Right Questions
Where can you find answers to those critical questions and get an early gauge as to how your AI investment is performing? By measuring the work that AI is impacting.
At its core, after all, AI is worker-focused technology, designed to help employees do their jobs more quickly, more easily, and more efficiently. But understanding whether things are actually playing out that way is about much more than simply learning how workers feel about their jobs and AI from a traditional employee experience survey.
What you need instead: ask questions that target the day-to-day activities that unfold in specific roles and detail the experience of actually doing the work. Topics for a software development team working with a new AI chatbot, for example, might include things like:
- How much time do you spend trying to find answers about the code base, on average, per week?
- Do you consider finding an answer about the code base under the current system to be easy or difficult?
- Does the new AI tool make finding answers in the code base easier or harder?
These types of specific questions aim to identify the touchpoints and moments that lead to work friction, which includes anything that gets in the way of a worker doing their job, including people, processes, and technology.
The goal is to uncover information about the work itself – not just employees’ feelings about that work – and to determine if it has been made better or worse by the introduction of an AI tool. Once you know where work friction is, you’ll have a better idea of where the ROI on your AI is likely to land.
Work Friction Is an Excellent Leading Indicator of AI ROI
Measuring work friction provides insight into whether an AI tool has had a positive impact on how employees are working, allowing you to validate an AI investment very early in the rollout. By looking specifically at employees’ task-by-task experiences and isolating the moments in their work days that slow them down or cause them trouble, you can get a clear picture of how AI is impacting those moments.
Comparing work friction data from before and after an AI implementation can offer early proof of productivity increases. But even decreases or new issues uncovered by the data can be useful, giving an organization a window into how its AI rollout is going – with specific areas to adjust if necessary.
For example, if you introduce an AI tool to speed up your software development process, it may take a while to see concrete evidence that it’s actually working; and if it isn’t, it may be hard to tell why.
With work friction data, however, your developers – the people actually interacting with the AI – will tell you how the technology is either helping or hurting their productivity. And if things aren’t going well, you’ll likely have some ideas of how to improve the AI implementation (instead of scrapping it altogether).
Even better, work friction data can give you an early idea of which of your more promising AI initiatives you might want to lean into. As former Grammarly CEO Rahul Roy-Chowdhury recently noted in a LinkedIn post about AI and ROI: “By continuously iterating and assessing your AI tools and use cases, you can cut through the clutter of AI promises and double down on what’s working.”
Don’t Use Old Methods to Measure A New Way of Working
Just as an AI transformation signals a new way of working, work friction represents a new way of measuring work. And in doing so, it serves as a way for leaders to get out ahead of any employee-related issues or problems that might derail their AI project.
In other words, amid growing pressure to prove ROI for an AI project, work friction can be a key early indicator of success or failure. By demonstrating how workers are adopting, adjusting to, and interacting with AI, an organization can have verifiable data to validate its investment and determine its future course of action – in weeks instead of years.
Related Resources
See all News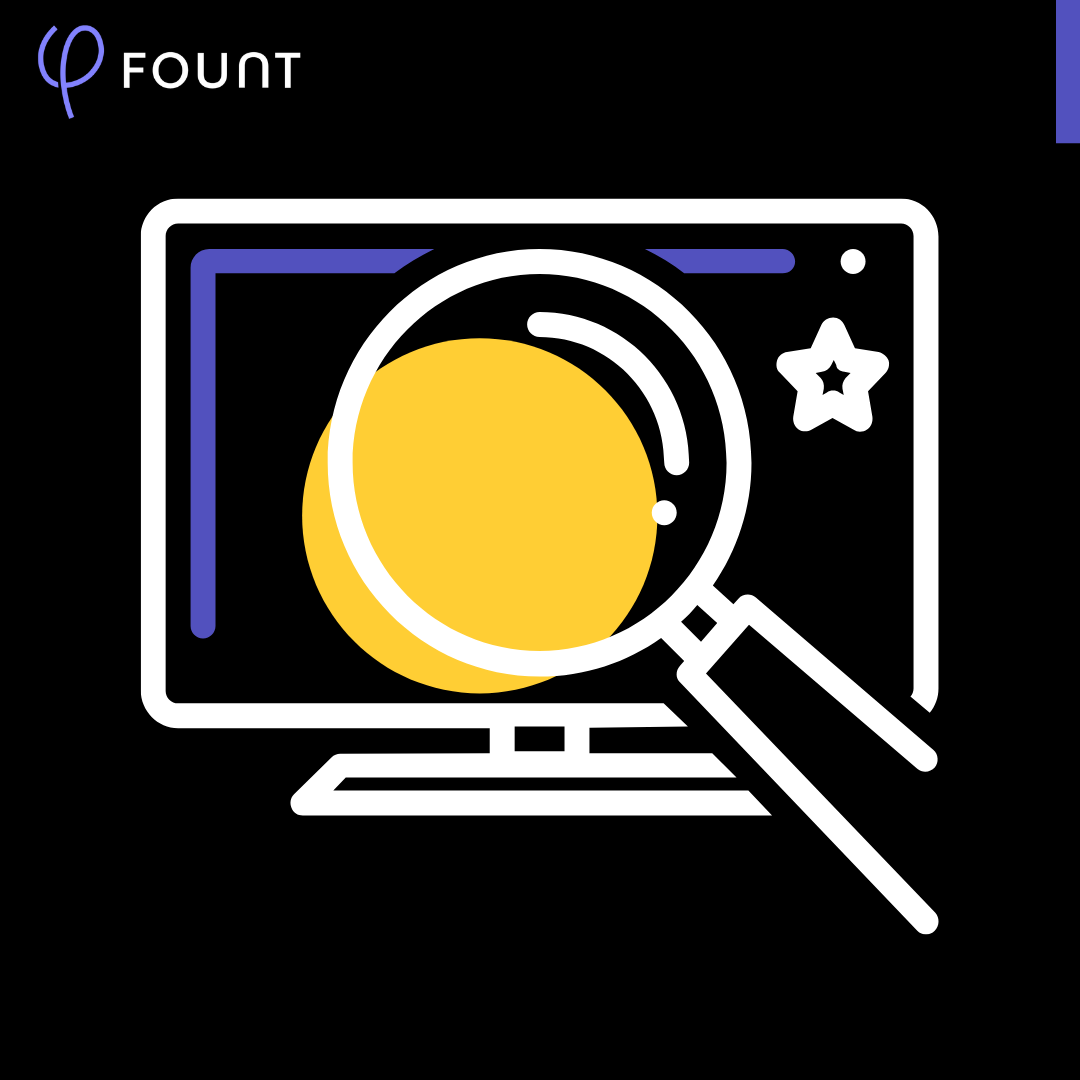
Insights
AI Implementations Need Better Validation Metrics
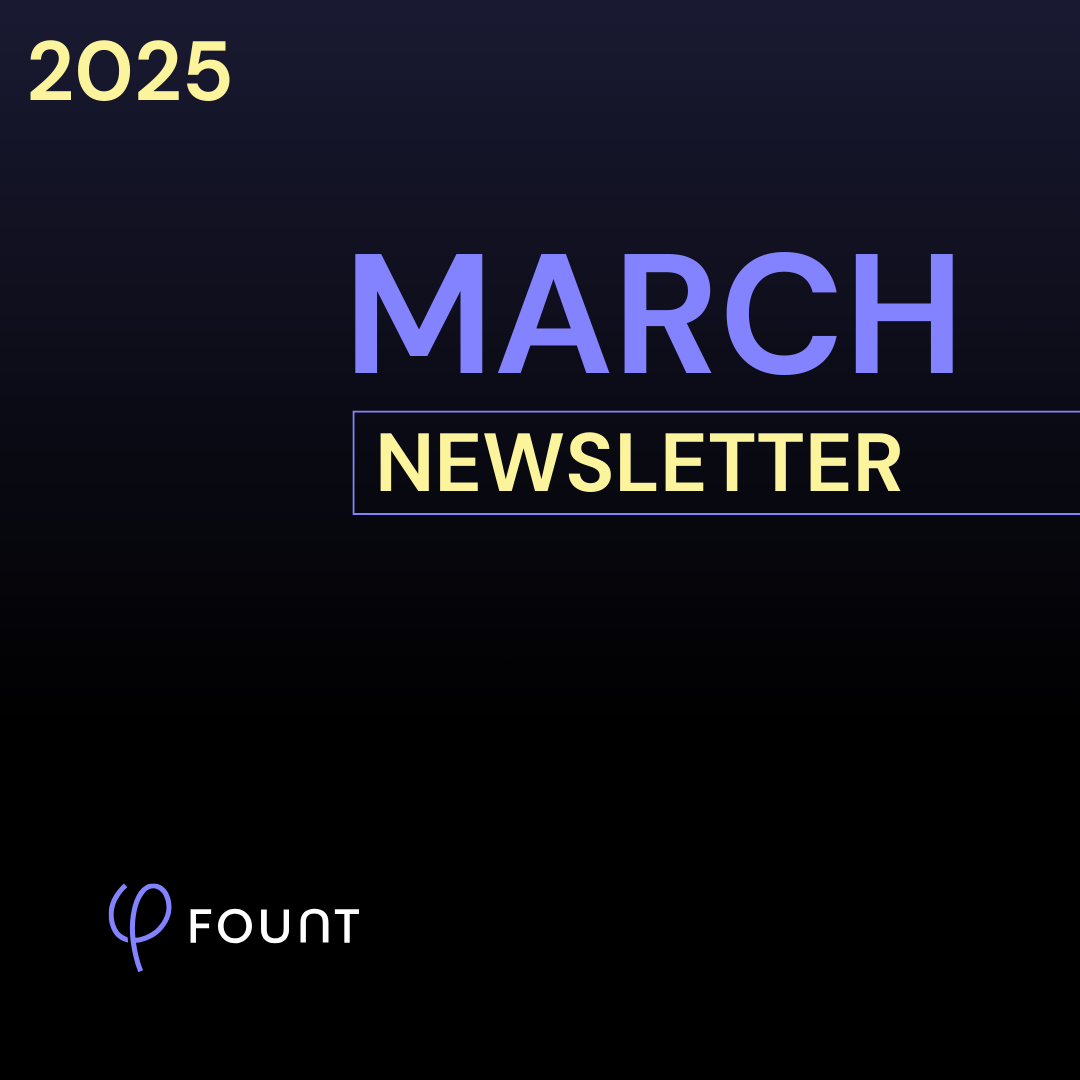
Insights
March Newsletter: Don’t Use Old Methods to Measure A New Way of Working
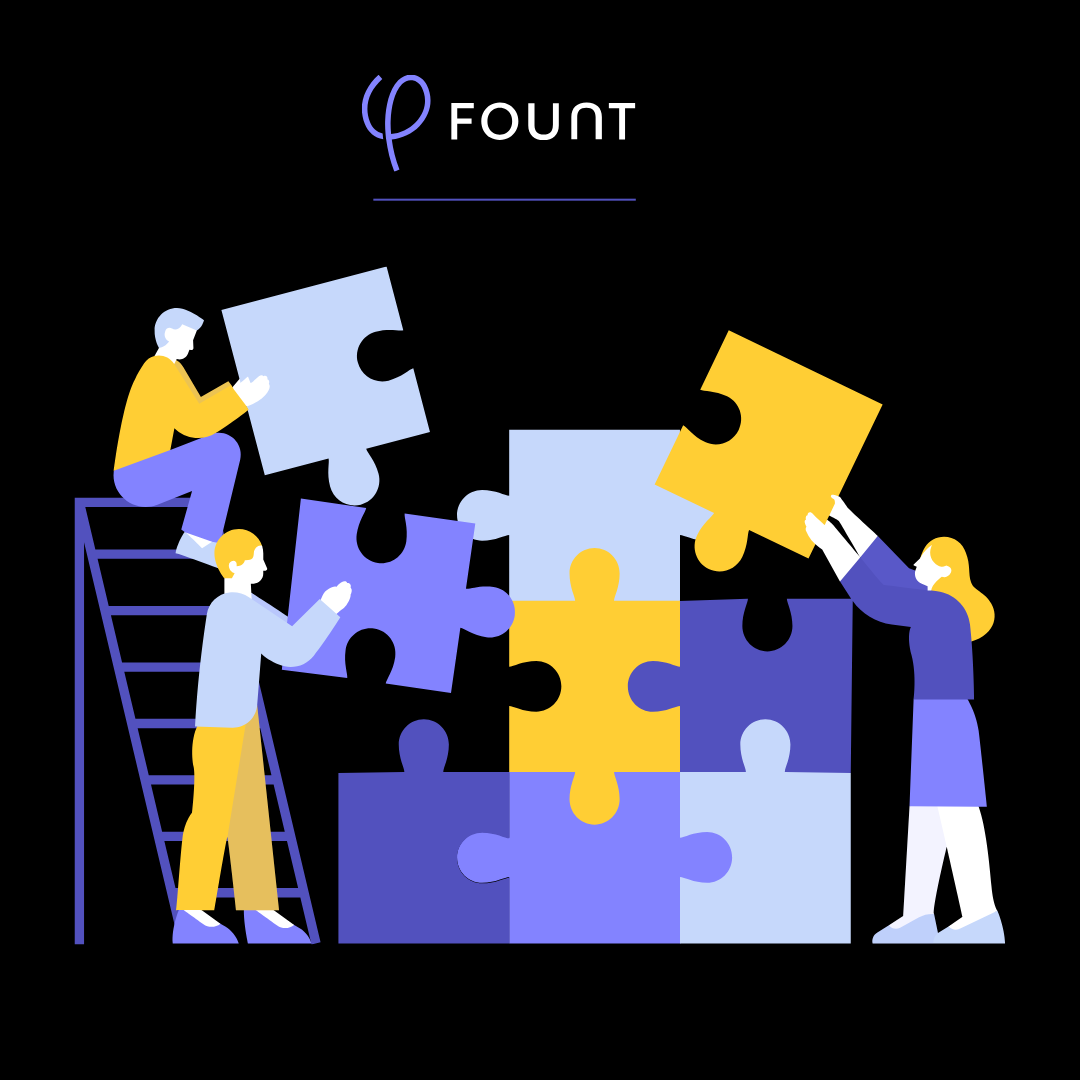
Insights
AI Transformation Playbook: The Definitive Guide to Measuring, Rescuing, Prioritizing, and Scaling AI Transformations
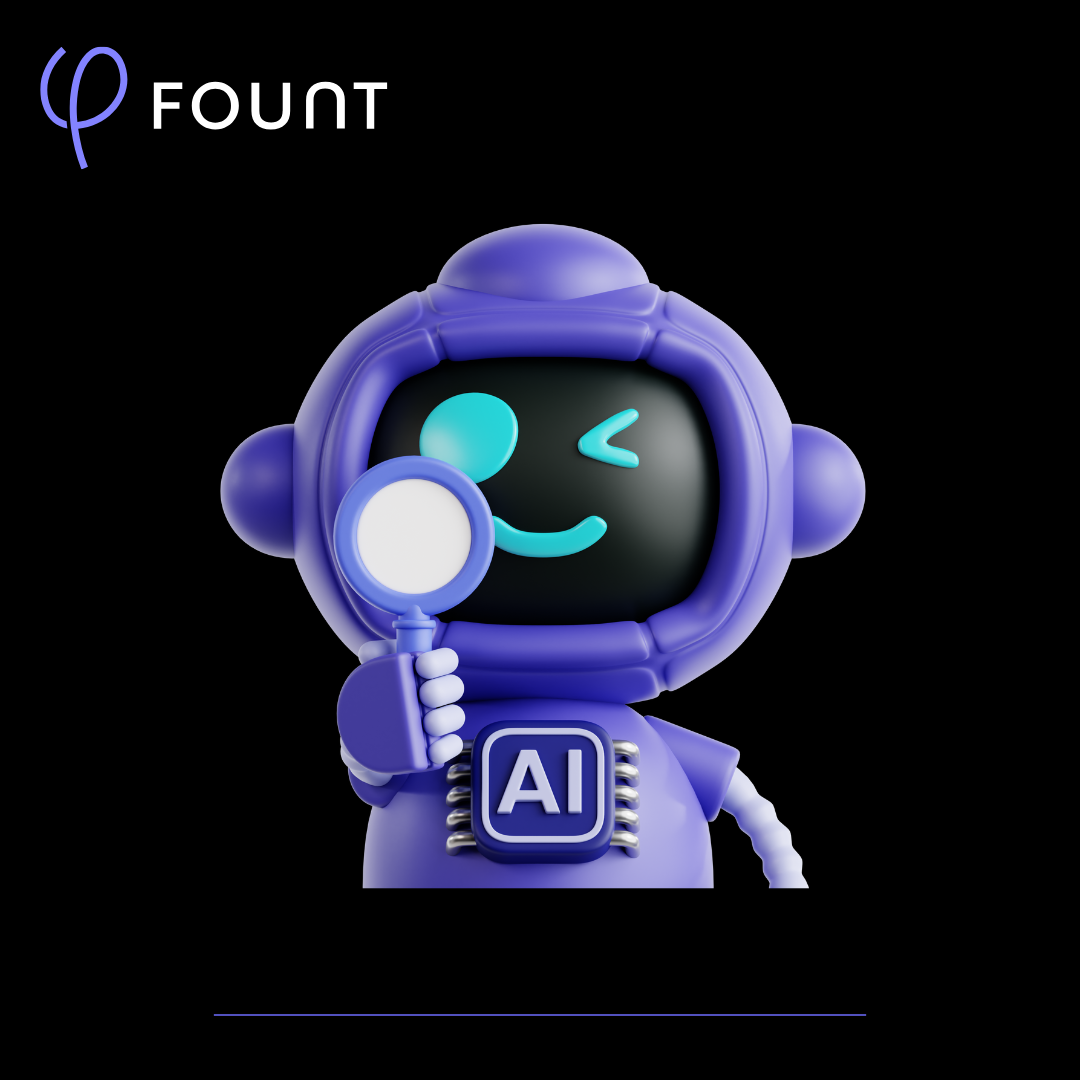
Insights
How AI Tools Change Your Team’s Work (And What to Do About It)
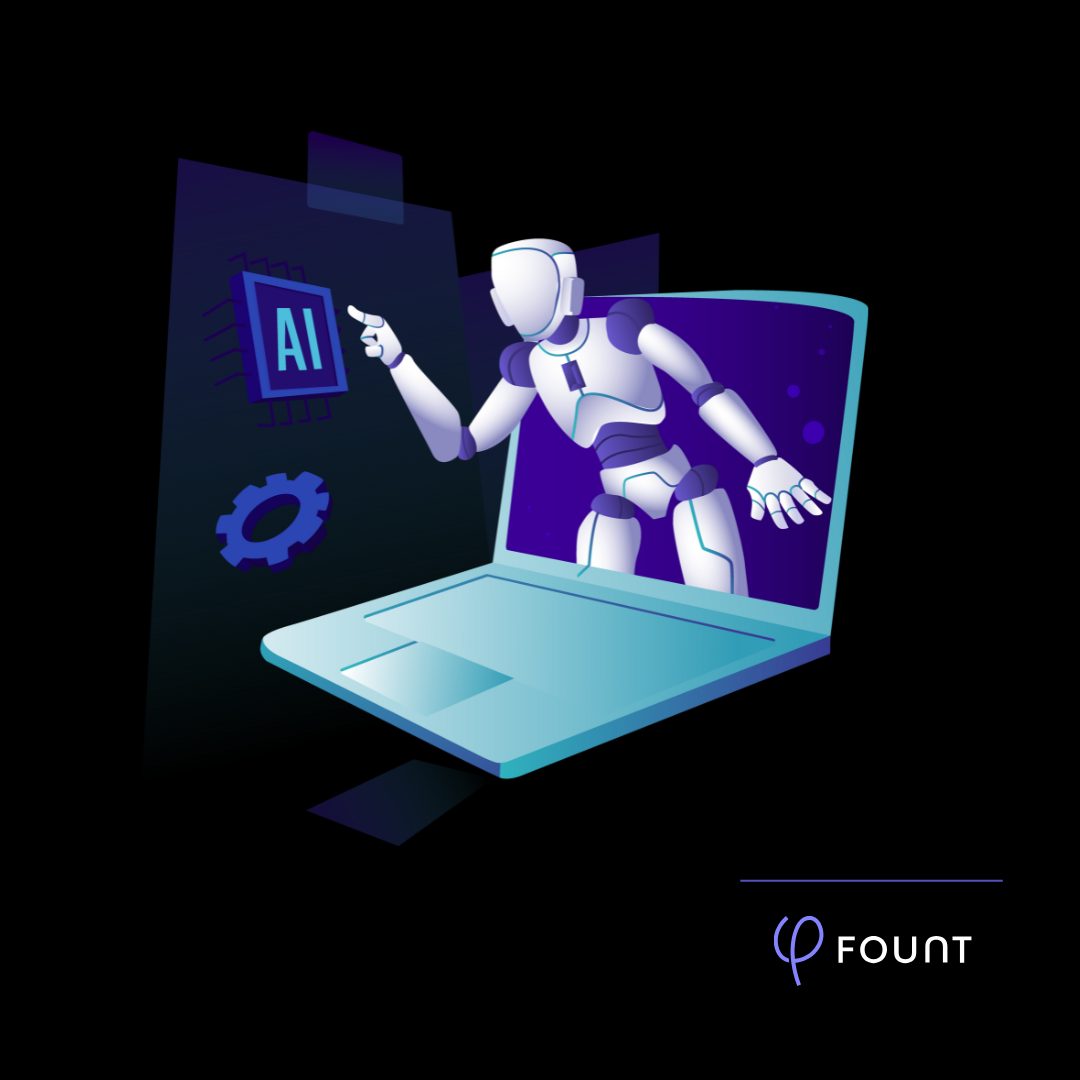
Insights
You Just Deployed a New AI Tool. How Soon Can You Know if It’s Working?
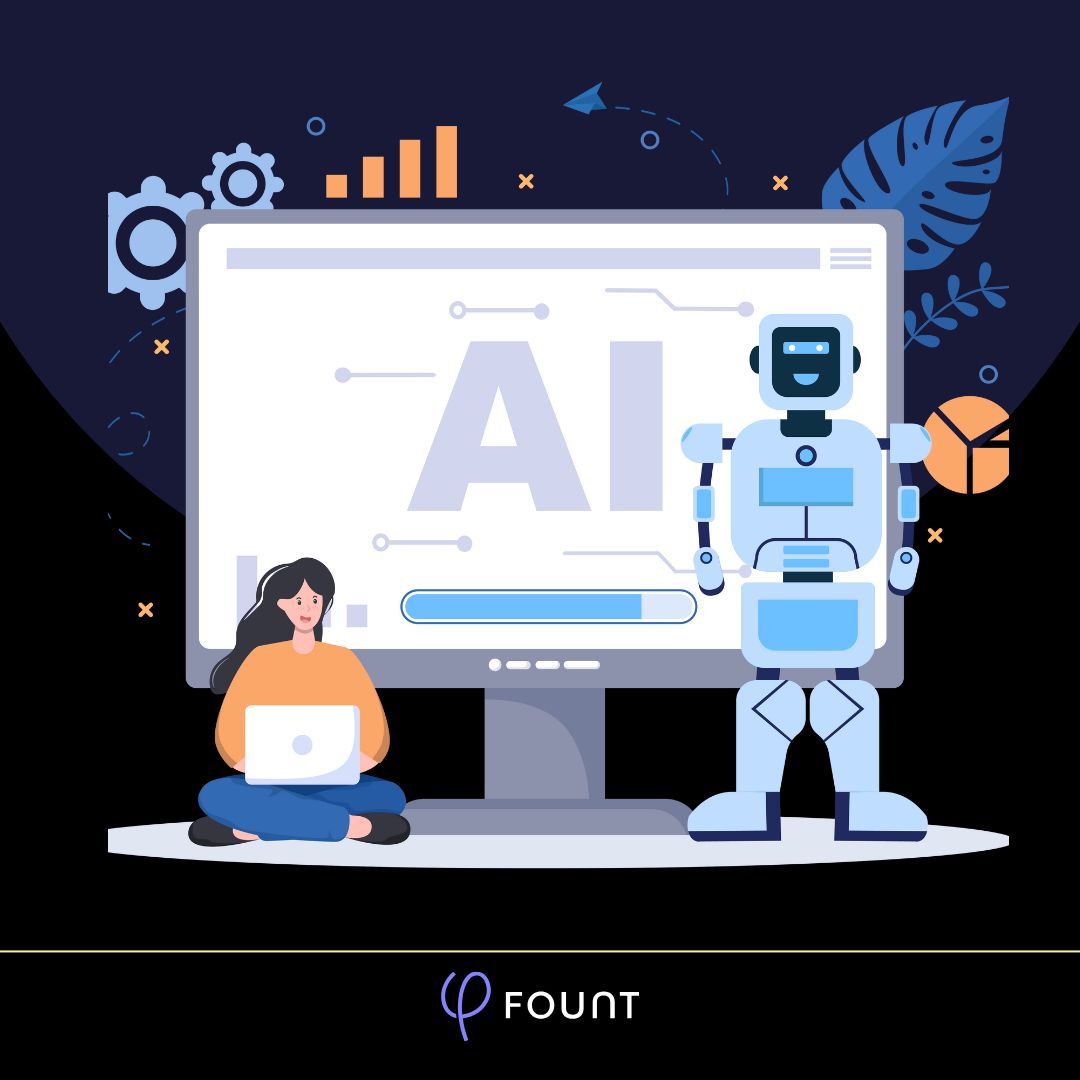
Insights
Worker Impact Is the Common Denominator of Every AI Transformation – And the Best Early Indicator of Success
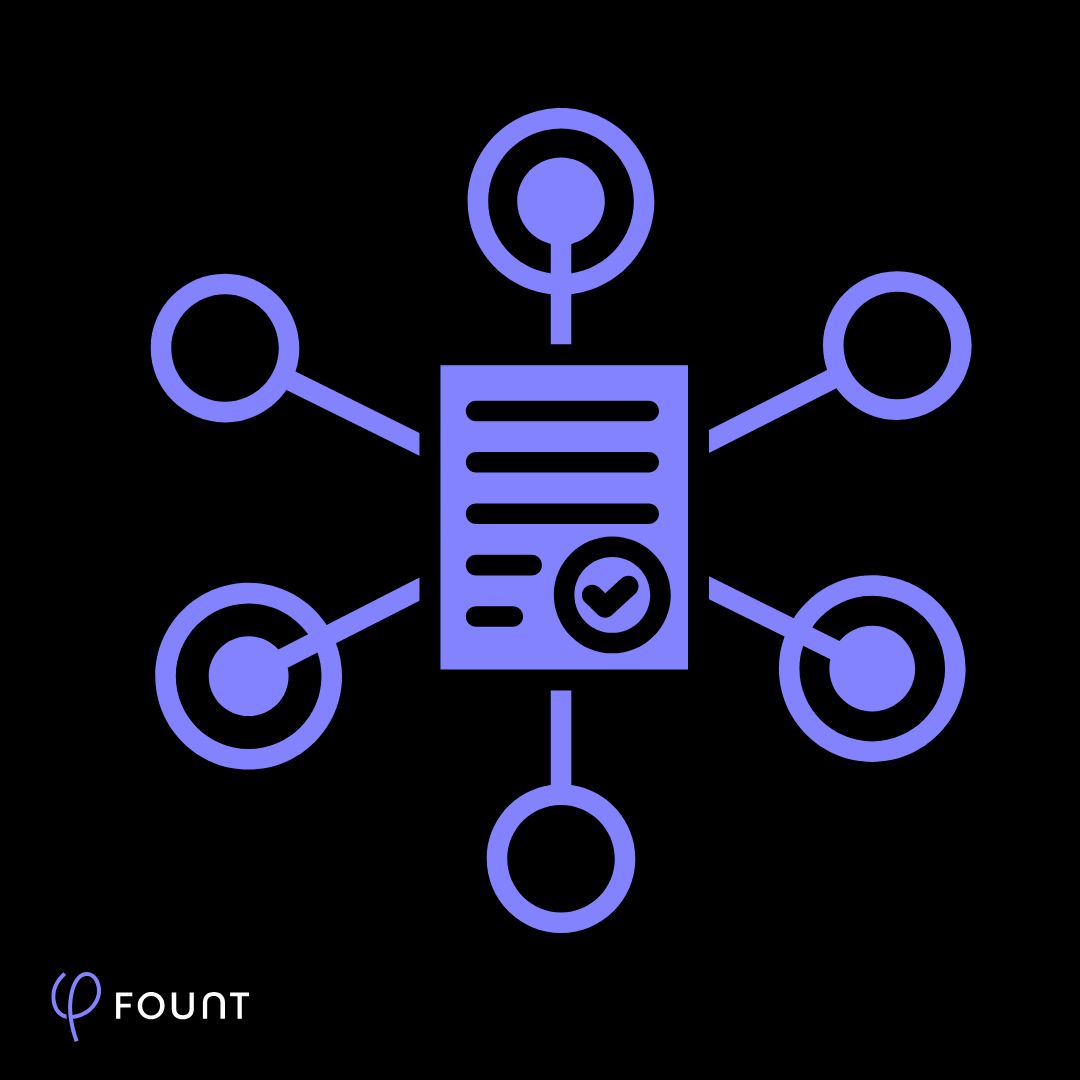
Insights
Data Deep Dive: The Origins of and Statistical Models Underpinning FOUNT’s Use of Data
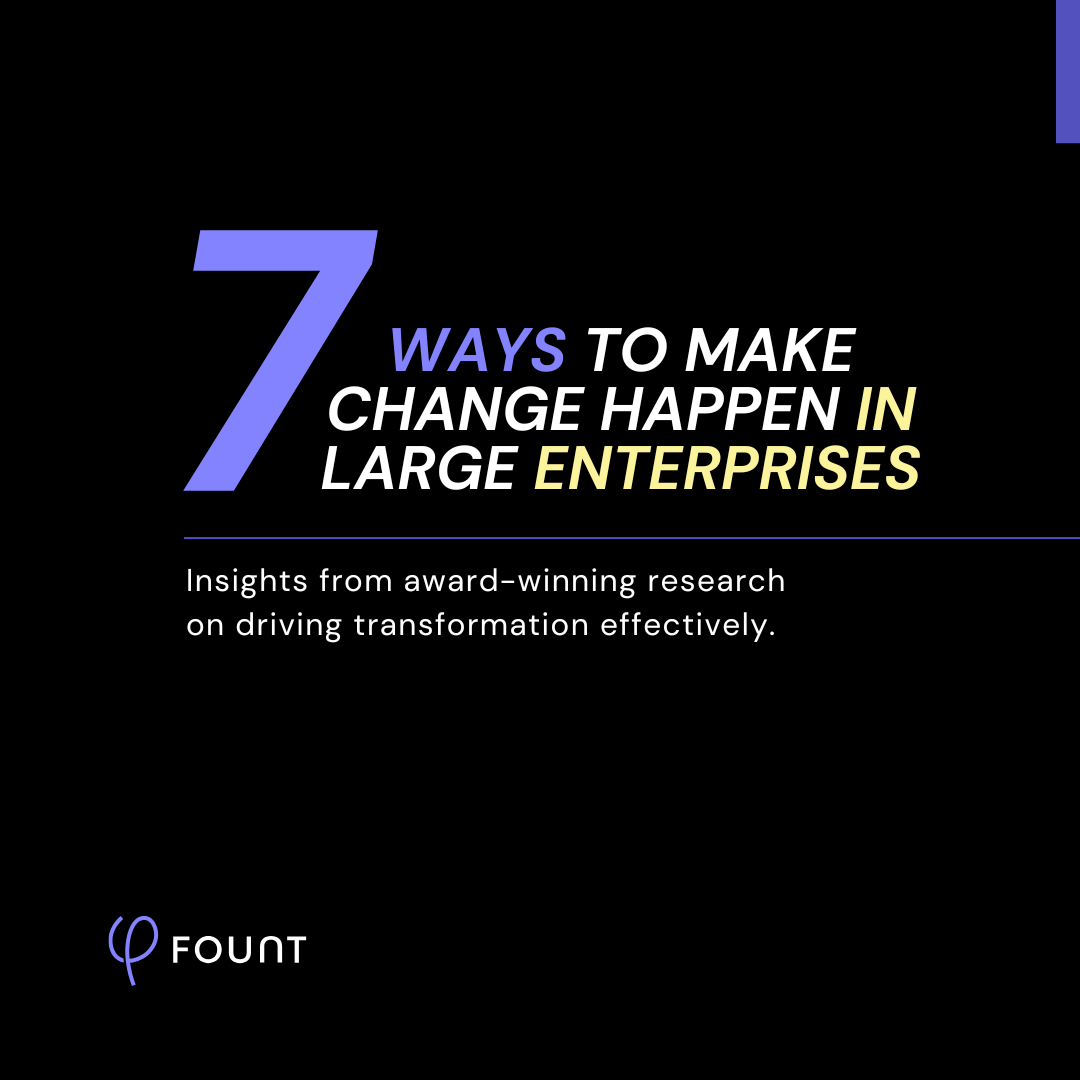
Guest Post
Original Research: 7 Ways to Make Change Happen in Large Enterprises